Postcards From The Data Edge

An electric vehicle driver adjusts control settings on the vehicle control screen.
By Vanessa Raymond
Feb. 17. 2023
Often what lies behind a dataset is a story, or a set of stories. Sometimes, it’s an epic saga complete with heroes, foes, trials and tribulations. Like all great stories, there are periods of woe and periods of triumph. Data stories are inextricable from human stories, with all the high drama that accompanies funding cycles, trends in research, and the crucial role that charismatic, passionate movers and shakers can have.
Working with data, as dry as that may sound to some, is actually quite an emotional process. Anyone who has spent any amount of time creating or collecting data, analyzing it, managing it, describing it, sharing, or re-using it can tell you how frustrating and exciting the process is. The challenges may stem from an ethical concern, a data quality concern, or a technical concern. Below read three data vignettes, accompanied by some bigger picture framing from data academics, featuring some of ACEP’s data champions: Michelle Wilber, Emily Richmond, and Dylan Palmieri.
Michelle Wilber, an ACEP Beneficial and Equitable Electrification researcher describes the care and caution she brings to her data work on electric vehicles in Alaska:
Most of the data that I play with is crowd-sourced electric vehicle (EV) data. In some cases, for example the Municipality of Anchorage’s electrical box truck data, which is a publicly-funded vehicle, the data is all public. Everyone involved in that project is signed on to making the data public, and so we have very little ethical concern or quandaries on a project like that.
The rest of the data I play with often comes from Alaskans who own and operate an EV and through the goodness of their heart share their data with me. When someone contributes their data to my research I have them sign a data sharing agreement, at which point I remove any personal info such as personally identifiable information (PII)… but even after all that we still have some challenging questions.
For example, when we mention a “Fairbanks Chevy Bolt” on a plot or graph about electric vehicles in Alaska. Well, as far as I know, there’s only one Chevy Bolt in Fairbanks. Even without the personally identifiable information, it’s still identifiable. Now most of what we are sharing from a data perspective is somewhat niche and esoteric; it’s published in a few papers and read by a handful of like-minded researchers. Mostly we’re talking about the impact of external temperature on trip efficiency, nothing overtly personal and not the hottest topic out there (literally!). Now if we had a broader audience for our data and our charts were being broadcast on the evening news, then that might be a different story.
Michelle describes how ACEP researchers, through their relationships and networks, maintain the trust of research partners and collaborations by protecting the people behind the data. Michelle also touches on the fact that when a larger spotlight is shone on research, the tools and techniques for managing the data may need to change to address the change in the scale of the interest, or the politicization of a research topic.
ACEP researchers seek to go above and beyond the requirements with their data relationships and networks, because, sometimes, behind the data we find the faintest whispers of peoples’ lives. Proceeding with caution, care, and empathy is the only just way to approach this. Wilber describes how even with powerhouse data, data that comes from an electric utility about the electrical output of the powerhouse, we can have a people-centric approach.
This situation illustrates how, even when we are using powerhouse data from communities we firmly believe that data belongs to the people who created it and the organization who shared it. Beyond just the personal protections, there’s the whole other challenge to ensure we are supporting energy sovereignty. At that level it’s not just personal data, it’s a relationship we try to make sure it’s respectful of another entity in total, be it a tribal council or community.
Michelle’s work echoes the thinking and cautioning of the biggest thinkers in data ethics, such as Kate Crawford, who speaks about the new harms introduced by big data and data science, challenging traditional research ethics guidelines:
Big Data stretches our concepts of ethical research in significant ways (Boyd and Crawford, 2012). It moves ethical inquiry away from traditional harms such as physical pain or a shortened lifespan to less tangible concepts such as information privacy impact and data discrimination. It may involve the traditional concept of a human subject as an individual, or it may affect a much wider distributed grouping or classification of people. It fundamentally changes our understanding of research data to be (at least in theory) infinitely connectable, indefinitely repurposable, continuously updatable and easily removed from the context of collection. By doing so, it forces us to grapple with the ways in which familiar and practical ethical constraints depend upon research data being temporally and contextually constrained and restricted by technical infrastructures and financial cost. Further, data science methods create an abstract relationship between researchers and subjects, where work is being done at a distant remove from the communities most concerned...1
Sometimes, the data story is just one of cleaning, or fixing. Like tinkerers outside of the data sphere, the headaches are numerous, unexpected, and can be quite vexing. ACEP’s data science analyst, Emily Richmond, is super annoyed with data right now. Why? Well she just discovered that some (but not all) of the data points she has been building an analysis off of are off by a decimal point. She vents because, to put it simply, she needs the story behind the data just as much as she needs the data itself.
We should be able to find this data online in a nice format, but no, it’s not that simple. It’s never that simple. We don’t have access to the source file [the original data collected that is then analyzed to create a published & final dataset], so we don’t know where the data came from. Why isn’t this data already public? It feels like someone is transcribing it, maybe some expert was making adjustments as they identified issues, but it makes it really hard to verify these numbers. I’m not an expert in the field so it’s hard for me to understand the difficulties they faced getting this data. I really wish they had some metadata, or source data files accompanying this dataset - it’s necessary for telling the story of the data so that we can use it. I feel like I'm floating between spreadsheets made by different people.
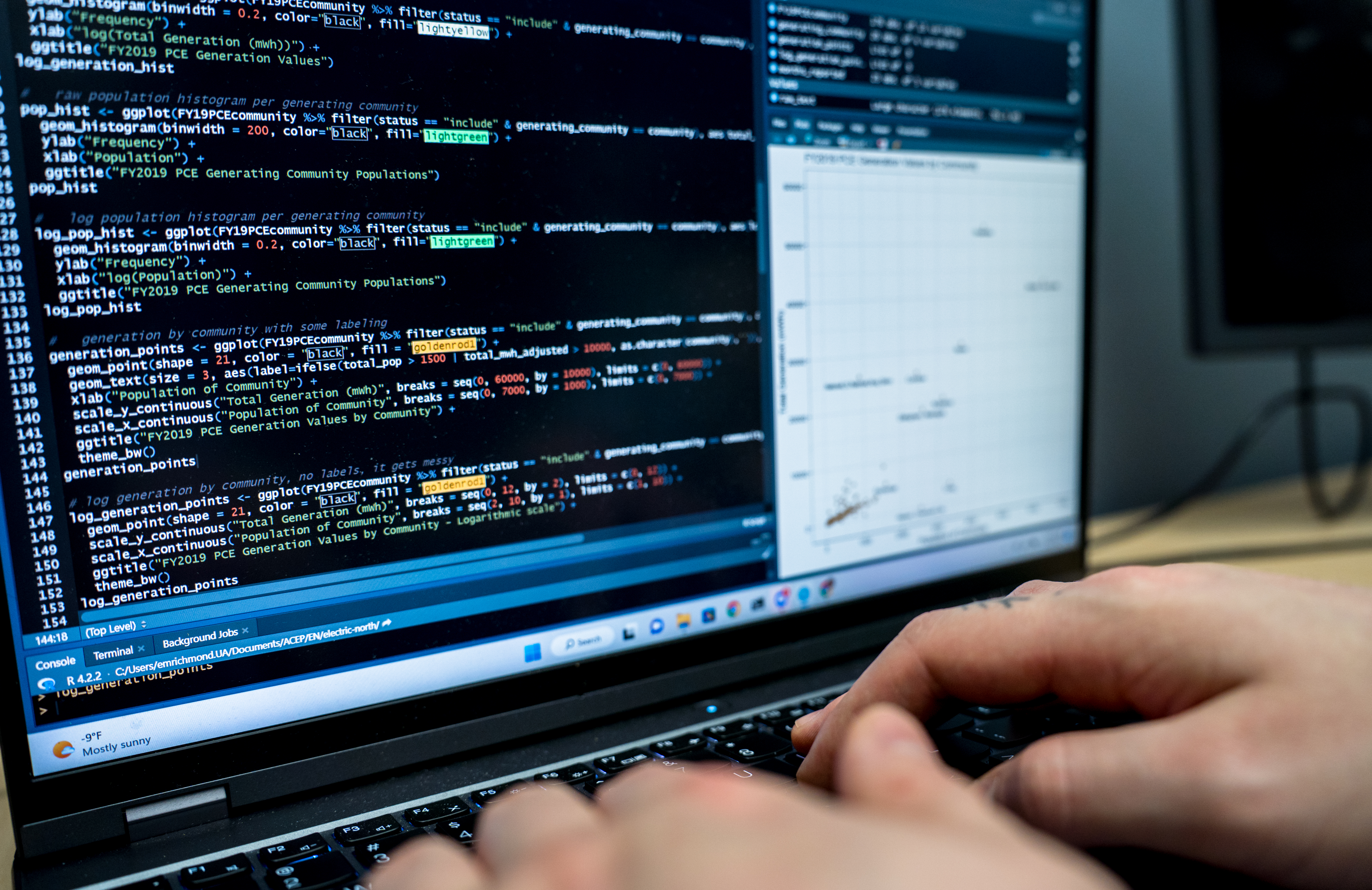
Emily Richmond, a data science analyst with ACEP's data collection and management team, generates custom power generation reports for communities across Alaska
Emily’s challenge is a familiar one in the data world. We inherit a dataset that’s incredibly valuable, however we don’t have the decoder ring - we can’t understand or verify how the data was made, that the data is accurate, and what decisions were made along the way to result in this dataset. Without good documentation, metadata, and some standard best practices being employed, it sometimes renders valuable data insights unusable for statistical analysis or other data science pursuits, just because of the high degree of uncertainty the data product introduces into the research process. The gold standard for datasets is that we receive a source or raw data file, the script that did the analysis, the data product that comes from the analysis, and we also receive metadata about the dataset: the who, what, when, where, and why, or the data’s origin story.
Mimi Onuoha is a data artist who has written at length about missing data, and in her 2018 essay “What is Missing Is Still There” 2 she attempts to define and describe data.
...academic Mitchell Whitelaw defines data as measurements extracted from the flux of the real. When we typically think of collecting data, we think of big important things: census information, UN data on health and diseases, data mined from large companies like Google, Amazon, or Facebook. From this perspective, Whitelaw’s definition of data is admirably concise and effective. With its clever use of the word “extraction”, it hints at the resource-driven nature of data collection… Whitelaw’s definition calls to mind corporate imaginings of data as a resource. In a capitalist society, it is always a smart business decision to collect data. A world collected is a world classified is a world rendered legible is a world made profitable. …a simpler definition comes to mind. Data: the things that we measure and care about…. Missing datasets is the term I have for these blank spots in a world that nowadays seems soaked in data. They form a ghostly parallel… they too are the facts of our world, the vertices of measurements. But they are the ones that we know little about. Data are what people care enough about to measure. Missing datasets are the things that people care about, but cannot measure.
Emily, in her work with ACEP, has chanced upon another type of data, the dataset that got forgotten. The data we used to care about, or perhaps that we used to be able to measure but can no longer.
ACEP’s Dylan Palmieri, Winter 2022 graduate of UAF’s computer science master's degree program, is tackling a different data challenge right now. Dylan’s challenge is one of documentation. It requires digging deep, really deep, into the way some air quality sensors were designed to understand the way the data is being created by the sensor. And if that wasn’t hard enough, he then needs to write code to translate this data into a format that ACEP researchers can easily understand in order to analyze the data coming out of the sensors.
“I basically want to write this software so that no one, and I mean no one, ever has to do what I have had to do” says Dylan. “I guess we can call it data democratization. It shouldn’t require a degree in computer science to draw conclusions from this data.” He goes on to describe his process further:
We start with the sensor documentation from the manufacturer. Yes, we read the manual! Actually I have read it four different times now. Then we look at the data outputs of the sensor. It’s not obvious what it all means. I realized I had to go deeper, and understand how the data gets created and structured to see how the sensor is creating the data. It’s “invisible work”, no one knows I had to go this far to be able to document the data. But, ultimately the researchers don’t want bits or bit strings, they want to see some numbers that make sense to them. I want this software and its associated documentation to abstract out the “niche” things and make it accessible to a general audience.
The day I figured out how this sensor worked was a good day. That was nice, that was fun. I enjoy the architecture work that comes with computer science and data work. I enjoy making pieces of code and software that you can string together to make a process. So I am creating a tool that translates the sensor data into something understandable, some format that the researchers care about. I am also making a few small tools, widgets of sorts, that analyze things for the researchers. I’d love one day to connect the sensor to the internet and create a way to stream data, but we’re not there yet. For now I am working on creating a dataset that really well describes itself, it has all the metadata [the data about the data] right there with the data. I want to write this documentation and craft the dataset interface so that for the majority of the people consuming it, it’s relatively intuitive.
Dylan’s work to fully understand a piece of hardware, write software and create data processes, and also document the way the data is structured and the outputs of the data is what makes research possible. It allows ACEP’s energy researchers and fuel cost research teams to focus on the analysis, bringing to bear all their subject matter expertise, to the research question at hand. This “invisible work” is also a time saving and cost saving measure that gets the data into a usable shape, without others also having to go down the rabbit hole later on.
Like Dylan, leading thinkers in data ethics, and specifically data feminists, are looking at this same concept of invisible work and data supply chains. Writes Catherine D’Ignazio and Lauren Klein in chapter “Show Your Work” from their 2020 book Data Feminism,
Coding is work, as anyone who’s ever programmed anything knows well. But it’s not always work that is easy to see. The same is true for collecting, analyzing, and visualizing data. We tend to marvel at the scale and complexity of an interactive visualization …But we are less often exposed to the networks of processes and people that help constitute the visualization itself…Unfortunately, however, when releasing a data product to the public, we tend not to credit the many hands who perform this work. We often cite the source of the dataset, and the names of the people who designed and implemented the code and graphic elements. But we rarely dig deeper to discover who created the data in the first place, who collected the data and processed them for use, and who else might have labored to make creations like the Ship Map possible. Admittedly, this information is sometimes hard to find. And when project teams (or individuals) are already operating at full capacity, or under budgetary strain, this information can—ironically—simply be too much additional work to pursue. Even in cases in which there are both resources and desire, information about the range of the contributors to any particular project sometimes can’t be found at all. But the various difficulties we encounter when trying to acknowledge this work reflects a larger problem in what information studies scholar Miriam Posner calls our data supply chain.
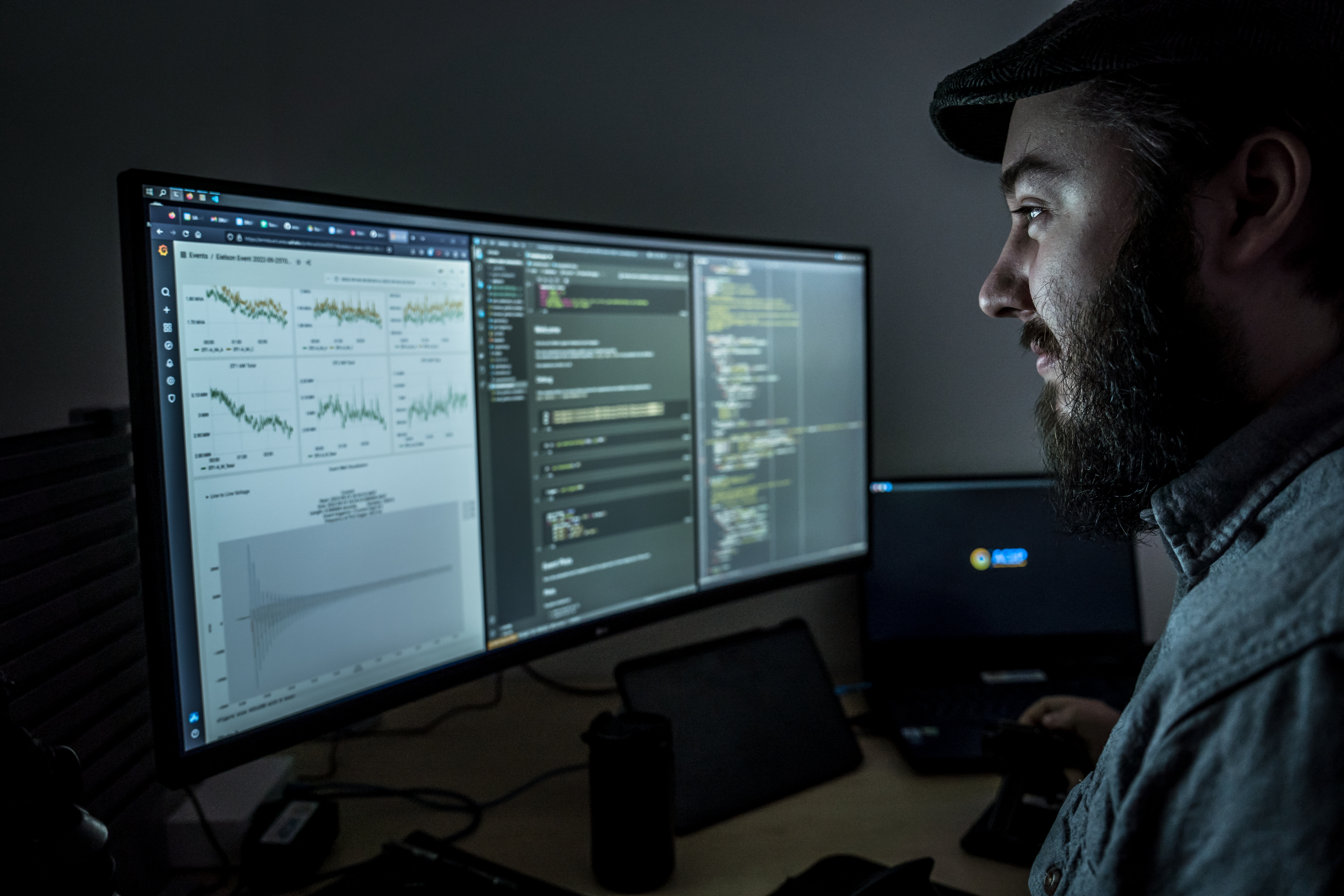
Dylan Palmieri of the ACEP data collection and management team analyzes power systems data on a visualization platform that he helped create.
The dangers in invisible work is if it is also siloed work, work that researchers, funders, community partners, or university stakeholders don’t see or understand. In this context, the invisible work can be underestimated in terms of time it takes to get from raw outputs from a sensor to a beautiful and compelling data visualization, in terms of the cost for clean and preparing data, and in terms of the skills and human capacity needed on a data-capable research team, to produce reliable data assets for research activities that solve some of Alaska’s most complex and pressing questions about the shape of our world today, and in the future.
ACEP enters 2023 with a deep commitment to Alaska’s energy data ecosystem that extends beyond individual grants and research projects to create a broader network of support of energy data in Alaska, one of the most unique and data rich energy landscapes in the nation. Under the direction of executive director Jeremy Kasper and executive officer Jennifer Harris, ACEP is poised to hire a cohort of data experts including devops system engineers who can create and maintain a data infrastructure that allows ACEP researchers to more easily receive and rely on data collected across remote communities in Alaska, and data science analysts like Emily Richmond who can support research teams at ACEP to clean and analyze data. In addition to creating these positions, ACEP is also launching a new initiative to have a data librarian internship project as part of the ACEP Undergraduate Summer Internship (AUSI) program, pilot a data club for high schoolers, and engage a cohort of computer science students in data, geospatial, and programming tasks related to energy data.
ACEP has also invested in a Data Governance Lead to facilitate the dynamic data rich environment of ACEP’s researchers. The data governance lead’s role is to create a culture and norms around data decisions at all levels of ACEP’s research enterprise to create some consistent, best practices for ethical and, where appropriate, accessible energy data products that benefit Alaskan remote communities, Alaskan energy researchers, and researchers around the world. As part of this effort, ACEP has joined the Interagency Arctic Research Policy Committee (IARPC) as co-chair of the Data Management team.
Data haikus
After the snow fall
data crunching hard like ice
How to melt and share
—Vanessa Raymond
Funding never lasts
Beyond nearest horizon
Halfway there will do
—Vanessa Raymond
data mgmt plan
required, confer
for a joyful exercise
—Vanessa Raymond
good, bad, dirty, flawed
wrangled, wrassled, abandoned
rescued, ninja'ed, qa'ed
—Vanessa Raymond
We just want to talk
There's no need to be afraid
It's all just numbers
—Emily Richmond
For we must muster
Up the obvious questions
To find the answers
—Emily Richmond
Where did it begin,
The minds of data design;
The means to my end
—Emily richmond
Data is vital
Accuracy is a must
Provides answers
—Alora Greer
Data, vast and deep
Endless streams of information
A world to explore
—openAI
Searching for meaning
Answers exist in data
Statistics blossom
—Dylan Palmieri
"a haiku", noted
Emily, "appears to have a
normal distribution"
—Kelsey Aho, US Forestry Service
b iological
i mpulsive events, or a
t hresholdy ʞɔɒd dılɟ
—Kelsey Aho, US Forestry Service
1 # do 'good' 'bad' labels
2 # remove the humanity
3 # from the data sets(?)
—Kelsey Aho, US Forestry Service
1 Metcalf, J., & Crawford, K. (2016). Where are human subjects in Big Data research? The emerging ethics divide. Big Data & Society 3(1). Read article online.
2 Onuoha, M. 2018. What is Missing is Still There. Nichons-Nous Dans L'Internet. Accessed at Download PDFversion of article.